When discussing about future scenarios, we figure out assumptions, boundaries and characteristics that could make such scenario to happen.
Related to this, the appearance of these future scenarios depends on signals: strong and weak signals.
A strong signal
is a phenomenon or thing that causes evident changes on the scenario. For instance, COVID-19 or a war is a strong signal that cannot be ignored and that all players will be identifying it and adjusting to it.
A weak signal
is a phenomenon or thing that can be an indicator of a potential change. It’s not as evident as a strong signal, but it could anticipate the existence of a future diffusion curve.
If you want to detect one of these weak signals, you have to look at the first adopters and place (if possible) sensors around them. In case you detect signals of change, you can anticipate and to get advantage of these signals.
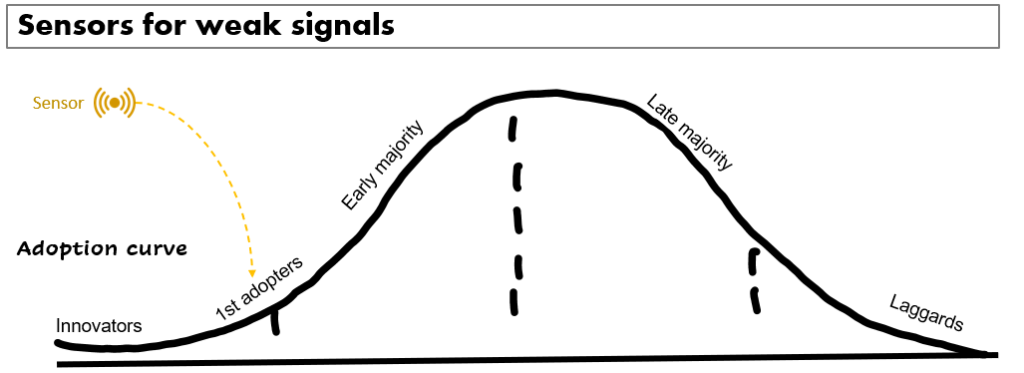
The position of sensors in other places is perfectly fine, the important thing is to understand where we are placing these sensors. In fact it’s very common to place sensors close to the borders of the stages.
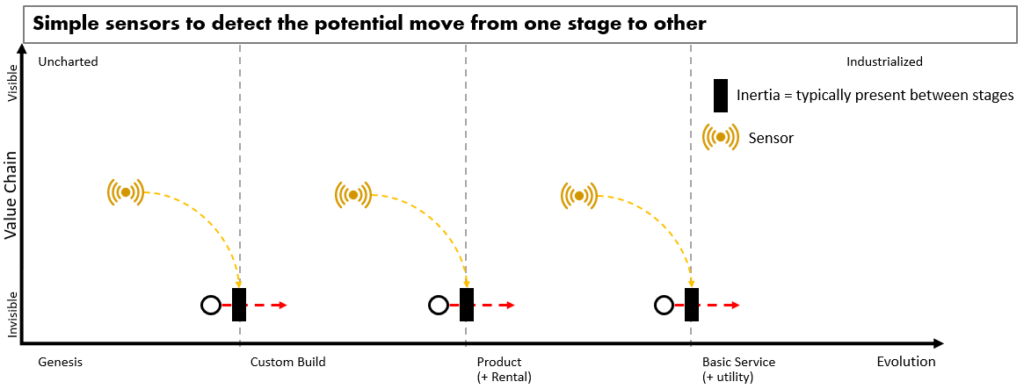
Evolution is different to diffusion
As the Wardley’s climatic pattern enunciates: Evolution consists of multiple waves of diffusion with many chasms.
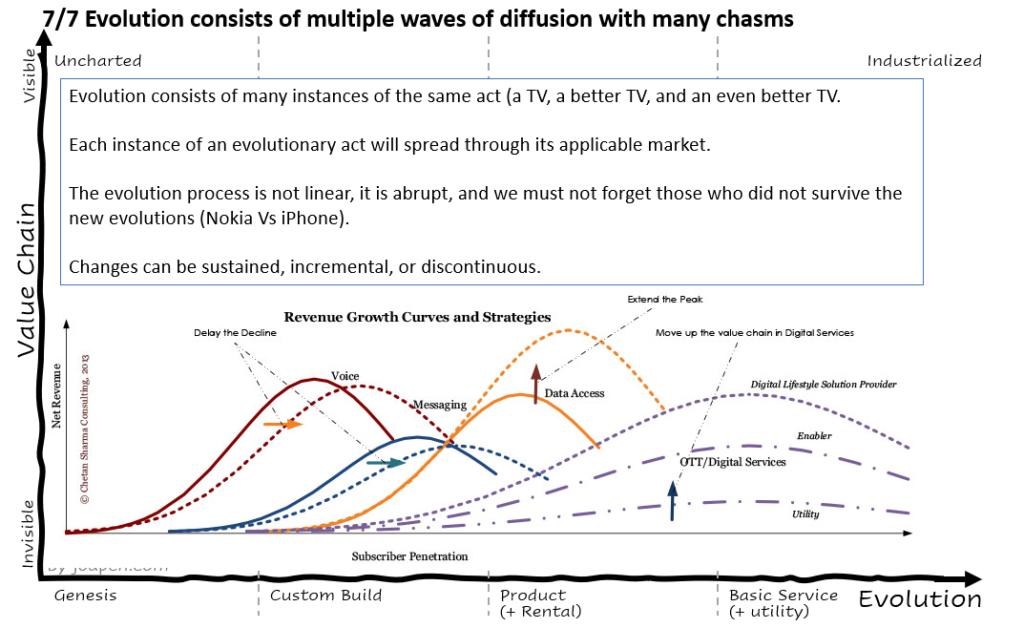
I want to highlight here, is that for weak signals, it’s very important:
- To figure out what diffusion curve we are expecting to happen,
- To figure out how other diffusion curves could interfere within our signal.
The art of placing sensors in the right place
When adding sensors that enable us to detect a potential phenomenon, we have to have context about where in our cycle these signals belong to, and who will be the beneficiary of the fact that the phenomenon is in deed happening.
For instance, in the context of digital product management; if we are mapping a product and we are testing the consumption of new features, we could add some sensors to detect the trend of consumption. The sensor itself needs to be positioned with respect the funnel we have for our product: as it is not the same to test a feature to attract new customers than a feature to try to increase the consumption of a premium user.
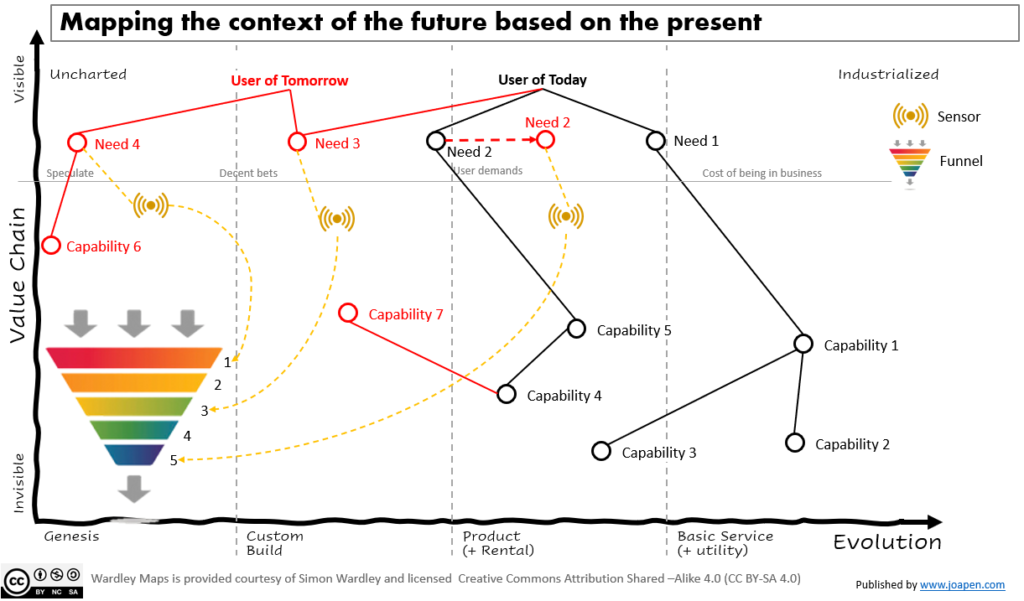
Note that I have added 2 users, the user of Today and the user of tomorrow. In this way I try to think about potential needs that are not an evolution of existing ones, and eliminate some bias.
When a sensor suddenly starts to give some positive data, we have to analyze the significance of the phenomenon, understand how close or how far is from the initial assumptions we did and decide what to do with these signal.
Collecting signals
First, I would like you to think as a Radio Control aficionado where you are willing to be in touch with some other people and you have limited technology to reach these long distance aficionados. This attitude sometimes help to put yourself in the right context.
Second, if you have access to a huge source of data, you could categorize all this data, for instance:
- PESTEC categorization (political, economic, social, technological, environmental and cultural signals),
- VERGE categorization (signals associated with defining, relating, interacting, production and consumption).
- STEEP framework (social, technological, economic, environmental, political).
You can be starving of data, and that could make you to fall into the “too many data” issue. Sometimes the simpler, the better.
Third, take into consideration that weak signals don’t usually fit neatly into one category: they are collectively exhaustive, not mutually exclusive.
Types of sensors
We can basically have two type of sensors: active and passive:
Passive sensors
- They are listeners, gather data that needs to be turned into convergent information.
Active sensors
- In E()Forward scout is a military term and its a way of finding out where the enemy is located. Send out lots of patrols see who doesn’t come back. So its an active form of weak signal detection.