There are different techniques or models to solve these type of problems. We can divide the type of models using this diagram:
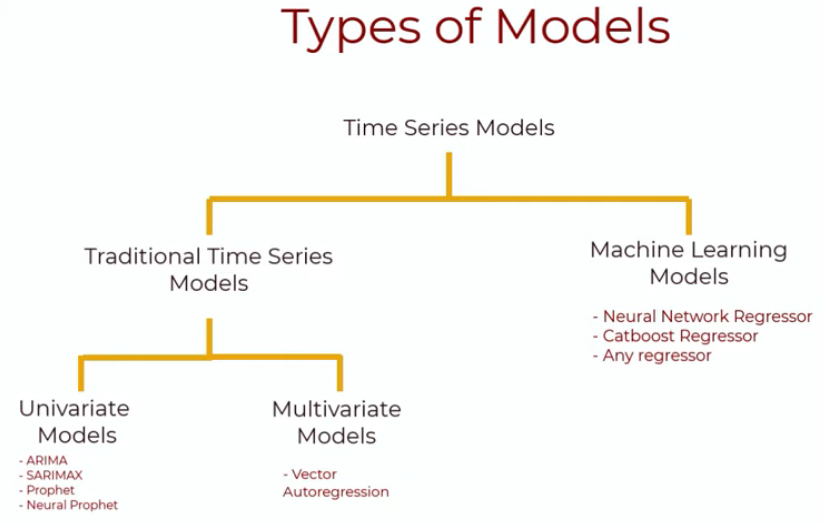
Traditional Time Series forecasting techniques
- Recursive: we can make predictions for tomorrow, the day after… to do the prediction of day #4 we will predict first #1, then #2, then #3 and then we will forecast #4.
- Easy to expand: if we want predictions for the next 10 days, just keep recursively making predictions.
- Thought to get right.
- Cannot add time varying features.
Examples of techniques:
- ARIMA
- SARIMA (seasonal ARIMA)
- Prophet
- Neural Prophet
- Vector Auto-regression (VAR)
Machine Learning models
- Direct: make predictions directly independently of the horizon.
- Thought to extend: training data increases linearly as we have more horizons to predict.
- Easy to get right.
- Can add time varying variables as features.
Example of techniques:
- Neural network regressor
- Catboost regressor
- Any regressor